POWER-INFORMED CYBERSECURITY
The DERSec solution is designed specifically to protect EV charger & solar+storage generation assets from network and power-system attacks. DERSec Sentry and DERSec Detective deliver coordinated fleet protection, increased uptime and higher asset availability for fleet operators.
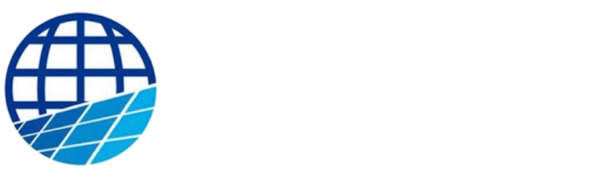
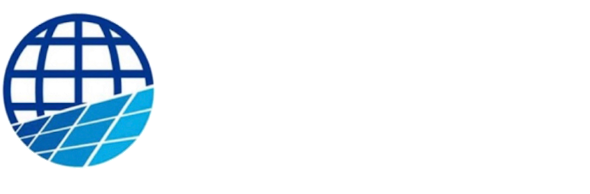
The industry’s first real-time, power-informed threat detection system for EV charging and solar+storage fleets. DERSec Sentry incorporates energy-protocol-specific deep packet inspection, patented power-signal anomaly detection, and machine learning to detect insider threats, malicious firmware updates, and improper local control system changes. DERSec Detective coordinates with DERSec Sentries to combat threats that impact asset availability and integrity across the fleet.
VALUE PROPOSITION
DETECTS POWER-BASED ATTACKS THAT OTHERS MISS
- Nefarious power control operations
- Malicious or faulty firmware updates
- Falsified monitoring data and other false data injection attacks
REVEALS AND STOPS LOCAL AND REMOTE INSIDER ATTACKS
- 64% of successful cyber attacks result from privileged user negligence
- 23% of attacks are perpetrated by malicious insiders
- Circumvents the most common type of adversary that is the hardest to catch
FAST, NON-INTRUSIVE, AND COMPREHENSIVE
- Deployed on hardened industrial compute devices and attached to a switch SPAN port (detection mode) or as a bump-in-the-wire (prevention mode)
- Security checks on all packets and local human interfaces completed in less than 500 milliseconds
- Complements existing network and security infrastructure, including fleet operator firewalls and Security Information And Event Management (SIEM) systems
OVERVIEW
DERSec Sentry and DERSec Detective work together to detect malicious firmware updates, nefarious control operations, and false data injection attacks on the network; learn adversarial tactics; increase grid resilience; and maximize asset uptime. It is the first solution in the market to detect power-based attacks that are perpetrated by insiders and/or nation-state actors on DER assets to commit crimes and destabilize the power grid.
HOW IT WORKS
DERSec Sentry and Detective both implement energy-informed Deep Packet Inspection technology to parse network traffic to extract monitoring and control signals and validate those signals against a set of power system rules. When falsified power data or malicious control signals are detected, DERSec Sentry and Detective suppress incoming network traffic and notify network operators of the activity.

THREAT COVERAGE

NETWORK CONFIGURATION
DERSec Sentry and DERSec Detective work together to address power-based threats to DER systems and the power grid that are perpetrated by insiders and others. DERSec Sentry is installed in DER and EV charging systems attached to a SPAN port for monitoring, threat detection, and threat protection. DERSec Detective is deployed in network operating centers of aggregators, DER vendors, and utility companies in front of the DER Management System (DERMS) to monitor network traffic, isolate and mitigate suspicious packets, and maintain network traffic flow.

DETECTION ALGORITHM
DERSec Deep Packet Inspection detection rulesets are applied to filter and drop packets before reaching the intended target, but with varying speeds of detection and accuracy. Customer-tunable heuristic rules that describe known-bad network operations can be applied at the packet level extremely quickly but cannot discern the operational state of the DER equipment. Stateful detection solves that problem by retaining operational information and detecting bad operations over longer timeframes. Digital twins go a step further and capture the complex interactions of multiple grid-support functions with the grid- and DC-power source states. Power simulation, updated with out-of-band and DER-control data, is run in parallel with the physical system to detect maloperations of the DER by tracking significant measurement deviations with the physical equipment. Finally, machine learning is used to classify DER measurements and control data using an offline learning methodology to capture attacks that are not detected using the previous techniques.
